Preparation for Group Assignment#
This tutorial contains various small guides for tasks you will need or come in handy in the upcoming group assignment.
We’re going to need a couple of packages for this tutorial:
from atlite.gis import ExclusionContainer
from atlite.gis import shape_availability
import atlite
from rasterio.plot import show
import geopandas as gpd
import pandas as pd
import xarray as xr
import matplotlib.pyplot as plt
import country_converter as coco
import atlite
Preparatory Downloads#
For this tutorial, we also need to download a few files, for which one can use the urllib
library:
Show code cell content
from urllib.request import urlretrieve
Show code cell content
fn = "era5-2013-NL.nc"
url = "https://tubcloud.tu-berlin.de/s/bAJj9xmN5ZLZQZJ/download/" + fn
urlretrieve(url, fn)
('era5-2013-NL.nc', <http.client.HTTPMessage at 0x7f5a202de050>)
Show code cell content
fn = "PROBAV_LC100_global_v3.0.1_2019-nrt_Discrete-Classification-map_EPSG-4326-NL.tif"
url = f"https://tubcloud.tu-berlin.de/s/567ckizz2Y6RLQq/download?path=%2Fcopernicus-glc&files={fn}"
urlretrieve(url, fn)
('PROBAV_LC100_global_v3.0.1_2019-nrt_Discrete-Classification-map_EPSG-4326-NL.tif',
<http.client.HTTPMessage at 0x7f5a2035a590>)
Show code cell content
fn = "WDPA_Oct2022_Public_shp-NLD.tif"
url = (
f"https://tubcloud.tu-berlin.de/s/567ckizz2Y6RLQq/download?path=%2Fwdpa&files={fn}"
)
urlretrieve(url, fn)
('WDPA_Oct2022_Public_shp-NLD.tif',
<http.client.HTTPMessage at 0x7f5a20360310>)
Show code cell content
fn = "GEBCO_2014_2D-NL.nc"
url = (
f"https://tubcloud.tu-berlin.de/s/567ckizz2Y6RLQq/download?path=%2Fgebco&files={fn}"
)
urlretrieve(url, fn)
('GEBCO_2014_2D-NL.nc', <http.client.HTTPMessage at 0x7f5a20362190>)
Downloading historical weather data from ERA5 with atlite
#
First, let’s load some small example country. Let’s say, the Netherlands.
fn = "https://tubcloud.tu-berlin.de/s/567ckizz2Y6RLQq/download?path=%2Fgadm&files=gadm_410-levels-ADM_1-NLD.gpkg"
regions = gpd.read_file(fn)
/opt/hostedtoolcache/Python/3.11.11/x64/lib/python3.11/site-packages/pyogrio/raw.py:198: RuntimeWarning: File /vsimem/pyogrio_fc70a2516b214ae9b393a12b795dfe34 has GPKG application_id, but non conformant file extension
return ogr_read(
regions.plot()
<Axes: >
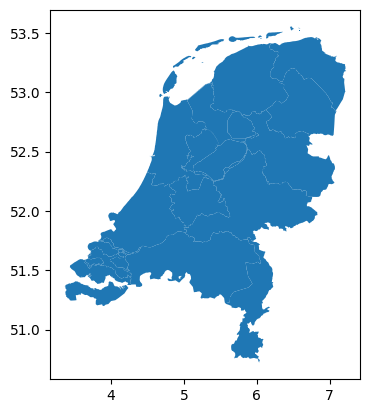
In this example we download historical weather data ERA5 data on-demand for a cutout we want to create.
Note
For this example to work, you should have
installed the Copernicus Climate Data Store
cdsapi
package (conda list cdsapi
orpip install cdsapi
) andregistered and setup your CDS API key as described on this website. Note that there are different instructions depending on your operating system.
A cutout is the basis for any of your work and calculations in atlite
.
The cutout is created in the directory and file specified by the relative path. If a cutout at the given location already exists, then this command will simply load the cutout again. If the cutout does not yet exist, it will specify the new cutout to be created.
For creating the cutout, you need to specify the dataset (e.g. ERA5), a time period and the spatial extent (in latitude and longitude).
minx, miny, maxx, maxy = regions.total_bounds
buffer = 0.25
cutout = atlite.Cutout(
path="era5-2013-NL.nc",
module="era5",
x=slice(minx - buffer, maxx + buffer),
y=slice(miny - buffer, maxy + buffer),
time="2013",
)
/opt/hostedtoolcache/Python/3.11.11/x64/lib/python3.11/site-packages/atlite/cutout.py:156: UserWarning: Arguments module, x, y, time are ignored, since cutout is already built.
warn(
Calling the function cutout.prepare()
initiates the download and processing of the weather data.
Because the download needs to be provided by the CDS servers, this might take a while depending on the amount of data requested.
Note
You can check the status of your request here.
# cutout.prepare(compression=None)
The data is accessible in cutout.data
. Included weather variables are listed in cutout.prepared_features
. Querying the cutout
gives us some basic information on which data is contained in it.
cutout.data
<xarray.Dataset> Size: 134MB Dimensions: (x: 17, y: 14, time: 8760) Coordinates: * x (x) float64 136B 3.25 3.5 3.75 4.0 ... 6.5 6.75 7.0 7.25 * y (y) float64 112B 50.5 50.75 51.0 ... 53.25 53.5 53.75 * time (time) datetime64[ns] 70kB 2013-01-01 ... 2013-12-31T23... lon (x) float64 136B dask.array<chunksize=(17,), meta=np.ndarray> lat (y) float64 112B dask.array<chunksize=(14,), meta=np.ndarray> Data variables: (12/13) height (y, x) float32 952B dask.array<chunksize=(14, 17), meta=np.ndarray> wnd100m (time, y, x) float32 8MB dask.array<chunksize=(100, 14, 17), meta=np.ndarray> wnd_azimuth (time, y, x) float32 8MB dask.array<chunksize=(100, 14, 17), meta=np.ndarray> roughness (time, y, x) float32 8MB dask.array<chunksize=(100, 14, 17), meta=np.ndarray> influx_toa (time, y, x) float32 8MB dask.array<chunksize=(100, 14, 17), meta=np.ndarray> influx_direct (time, y, x) float32 8MB dask.array<chunksize=(100, 14, 17), meta=np.ndarray> ... ... albedo (time, y, x) float32 8MB dask.array<chunksize=(100, 14, 17), meta=np.ndarray> solar_altitude (time, y, x) float64 17MB dask.array<chunksize=(100, 14, 17), meta=np.ndarray> solar_azimuth (time, y, x) float64 17MB dask.array<chunksize=(100, 14, 17), meta=np.ndarray> temperature (time, y, x) float64 17MB dask.array<chunksize=(100, 14, 17), meta=np.ndarray> soil temperature (time, y, x) float64 17MB dask.array<chunksize=(100, 14, 17), meta=np.ndarray> runoff (time, y, x) float32 8MB dask.array<chunksize=(100, 14, 17), meta=np.ndarray> Attributes: module: era5 prepared_features: ['influx', 'wind', 'height', 'temperature', 'runoff'] chunksize_time: 100 Conventions: CF-1.6 history: 2023-01-15 21:33:09 GMT by grib_to_netcdf-2.25.1: /op...
cutout.prepared_features
module feature
era5 height height
wind wnd100m
wind wnd_azimuth
wind roughness
influx influx_toa
influx influx_direct
influx influx_diffuse
influx albedo
influx solar_altitude
influx solar_azimuth
temperature temperature
temperature soil temperature
runoff runoff
dtype: object
cutout
<Cutout "era5-2013-NL">
x = 3.25 ⟷ 7.25, dx = 0.25
y = 50.50 ⟷ 53.75, dy = 0.25
time = 2013-01-01 ⟷ 2013-12-31, dt = h
module = era5
prepared_features = ['height', 'wind', 'influx', 'temperature', 'runoff']
Repetition: From Land Eligibility Analysis to Availability Matrix#
We’re going to use the plotting functions from previous exercises:
def plot_area(masked, transform, shape):
fig, ax = plt.subplots(figsize=(5, 5))
ax = show(masked, transform=transform, cmap="Greens", vmin=0, ax=ax)
shape.plot(ax=ax, edgecolor="k", color="None", linewidth=1)
First, we collect all exclusion and inclusion criteria in an ExclusionContainer
.
excluder = ExclusionContainer(crs=3035, res=300)
fn = "PROBAV_LC100_global_v3.0.1_2019-nrt_Discrete-Classification-map_EPSG-4326-NL.tif"
excluder.add_raster(fn, codes=[50], buffer=1000, crs=4326)
excluder.add_raster(fn, codes=[20, 30, 40, 60], crs=4326, invert=True)
fn = "WDPA_Oct2022_Public_shp-NLD.tif"
excluder.add_raster(fn, crs=3035)
fn = "GEBCO_2014_2D-NL.nc"
excluder.add_raster(fn, codes=lambda x: x > 10, crs=4326, invert=True)
Then, we can calculate the available areas…
masked, transform = shape_availability(regions.to_crs(3035).geometry, excluder)
… and plot it:
plot_area(masked, transform, regions.to_crs(3035).geometry)
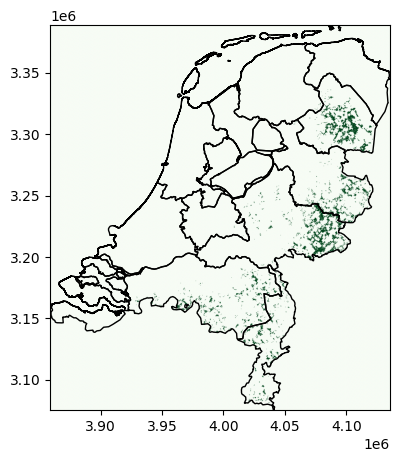
Availability Matrix#
regions.index = regions.GID_1
cutout = atlite.Cutout("era5-2013-NL.nc")
?cutout.availabilitymatrix
A = cutout.availabilitymatrix(regions, excluder)
cap_per_sqkm = 1.7 # MW/km2
area = cutout.grid.set_index(["y", "x"]).to_crs(3035).area / 1e6
area = xr.DataArray(area, dims=("spatial"))
capacity_matrix = A.stack(spatial=["y", "x"]) * area * cap_per_sqkm
Solar PV Profiles#
pv = cutout.pv(
panel=atlite.solarpanels.CdTe,
matrix=capacity_matrix,
orientation="latitude_optimal",
index=regions.index,
per_unit=True,
)
pv.to_pandas().head()
GID_1 | NLD.1_1 | NLD.2_1 | NLD.3_1 | NLD.4_1 | NLD.5_1 | NLD.6_1 | NLD.7_1 | NLD.8_1 | NLD.9_1 | NLD.10_1 | NLD.11_1 | NLD.12_1 | NLD.13_1 | NLD.14_1 |
---|---|---|---|---|---|---|---|---|---|---|---|---|---|---|
time | ||||||||||||||
2013-01-01 00:00:00 | 0.0 | 0.0 | 0.0 | 0.0 | 0.0 | 0.0 | 0.0 | 0.0 | 0.0 | 0.0 | 0.0 | 0.0 | 0.0 | 0.0 |
2013-01-01 01:00:00 | 0.0 | 0.0 | 0.0 | 0.0 | 0.0 | 0.0 | 0.0 | 0.0 | 0.0 | 0.0 | 0.0 | 0.0 | 0.0 | 0.0 |
2013-01-01 02:00:00 | 0.0 | 0.0 | 0.0 | 0.0 | 0.0 | 0.0 | 0.0 | 0.0 | 0.0 | 0.0 | 0.0 | 0.0 | 0.0 | 0.0 |
2013-01-01 03:00:00 | 0.0 | 0.0 | 0.0 | 0.0 | 0.0 | 0.0 | 0.0 | 0.0 | 0.0 | 0.0 | 0.0 | 0.0 | 0.0 | 0.0 |
2013-01-01 04:00:00 | 0.0 | 0.0 | 0.0 | 0.0 | 0.0 | 0.0 | 0.0 | 0.0 | 0.0 | 0.0 | 0.0 | 0.0 | 0.0 | 0.0 |
pv.to_pandas().iloc[:, 0].plot()
<Axes: xlabel='time'>
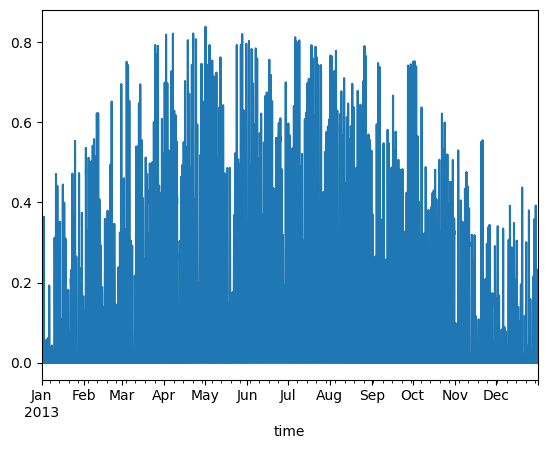
Wind Profiles#
wind = cutout.wind(
atlite.windturbines.Vestas_V112_3MW,
matrix=capacity_matrix,
index=regions.index,
per_unit=True,
)
/opt/hostedtoolcache/Python/3.11.11/x64/lib/python3.11/site-packages/atlite/resource.py:90: FutureWarning: 'add_cutout_windspeed' for wind turbine
power curves will default to True in atlite relase v0.2.15.
warnings.warn(msg, FutureWarning)
wind.to_pandas().head()
GID_1 | NLD.1_1 | NLD.2_1 | NLD.3_1 | NLD.4_1 | NLD.5_1 | NLD.6_1 | NLD.7_1 | NLD.8_1 | NLD.9_1 | NLD.10_1 | NLD.11_1 | NLD.12_1 | NLD.13_1 | NLD.14_1 |
---|---|---|---|---|---|---|---|---|---|---|---|---|---|---|
time | ||||||||||||||
2013-01-01 00:00:00 | 0.999056 | 0.998485 | 0.998294 | 0.997185 | 0.998198 | 0.998937 | 0.997705 | 0.998457 | 0.996653 | 0.998057 | 0.996010 | 0.998684 | 0.997938 | 0.995548 |
2013-01-01 01:00:00 | 0.991667 | 0.993051 | 0.970360 | 0.995386 | 0.953421 | 0.994112 | 0.997151 | 0.997635 | 0.984541 | 0.997531 | 0.985574 | 0.996537 | 0.992089 | 0.983092 |
2013-01-01 02:00:00 | 0.981956 | 0.970941 | 0.933896 | 0.982949 | 0.903412 | 0.974296 | 0.995252 | 0.993278 | 0.939996 | 0.989856 | 0.956938 | 0.968682 | 0.976204 | 0.952877 |
2013-01-01 03:00:00 | 0.948241 | 0.870216 | 0.846933 | 0.946690 | 0.840527 | 0.877124 | 0.980648 | 0.951256 | 0.773720 | 0.957361 | 0.852354 | 0.853057 | 0.899308 | 0.837002 |
2013-01-01 04:00:00 | 0.770508 | 0.647249 | 0.572062 | 0.846147 | 0.593890 | 0.611827 | 0.921734 | 0.850735 | 0.490069 | 0.841523 | 0.681832 | 0.705750 | 0.730653 | 0.623015 |
wind.to_pandas().iloc[:, 0].plot()
<Axes: xlabel='time'>
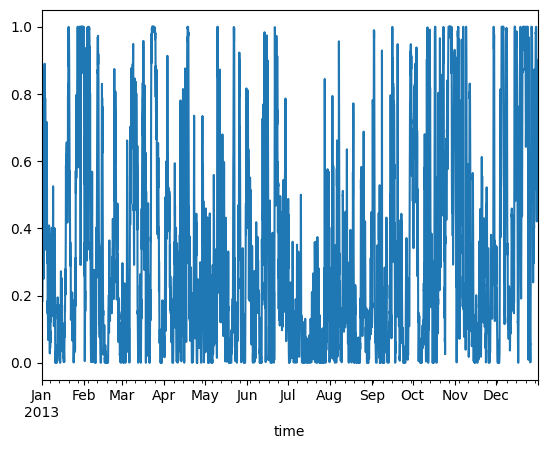
Merging Shapes in geopandas
#
Spatial data is often more granular than we need. For example, we might have data on sub-national units, but we’re actually interested in studying patterns at the level of countries.
Whereas in pandas
we would use the groupby()
function to aggregate entries, in geopandas
, we aggregate geometric features using the dissolve()
function.
Suppose we are interested in studying continents, but we only have country-level data like the country dataset included in geopandas
. We can easily convert this to a continent-level dataset.
world = gpd.read_file("https://naciscdn.org/naturalearth/110m/cultural/ne_110m_admin_0_countries.zip")
world.head(3)
featurecla | scalerank | LABELRANK | SOVEREIGNT | SOV_A3 | ADM0_DIF | LEVEL | TYPE | TLC | ADMIN | ... | FCLASS_TR | FCLASS_ID | FCLASS_PL | FCLASS_GR | FCLASS_IT | FCLASS_NL | FCLASS_SE | FCLASS_BD | FCLASS_UA | geometry | |
---|---|---|---|---|---|---|---|---|---|---|---|---|---|---|---|---|---|---|---|---|---|
0 | Admin-0 country | 1 | 6 | Fiji | FJI | 0 | 2 | Sovereign country | 1 | Fiji | ... | None | None | None | None | None | None | None | None | None | MULTIPOLYGON (((180 -16.06713, 180 -16.55522, ... |
1 | Admin-0 country | 1 | 3 | United Republic of Tanzania | TZA | 0 | 2 | Sovereign country | 1 | United Republic of Tanzania | ... | None | None | None | None | None | None | None | None | None | POLYGON ((33.90371 -0.95, 34.07262 -1.05982, 3... |
2 | Admin-0 country | 1 | 7 | Western Sahara | SAH | 0 | 2 | Indeterminate | 1 | Western Sahara | ... | Unrecognized | Unrecognized | Unrecognized | None | None | Unrecognized | None | None | None | POLYGON ((-8.66559 27.65643, -8.66512 27.58948... |
3 rows × 169 columns
world
featurecla | scalerank | LABELRANK | SOVEREIGNT | SOV_A3 | ADM0_DIF | LEVEL | TYPE | TLC | ADMIN | ... | FCLASS_TR | FCLASS_ID | FCLASS_PL | FCLASS_GR | FCLASS_IT | FCLASS_NL | FCLASS_SE | FCLASS_BD | FCLASS_UA | geometry | |
---|---|---|---|---|---|---|---|---|---|---|---|---|---|---|---|---|---|---|---|---|---|
0 | Admin-0 country | 1 | 6 | Fiji | FJI | 0 | 2 | Sovereign country | 1 | Fiji | ... | None | None | None | None | None | None | None | None | None | MULTIPOLYGON (((180 -16.06713, 180 -16.55522, ... |
1 | Admin-0 country | 1 | 3 | United Republic of Tanzania | TZA | 0 | 2 | Sovereign country | 1 | United Republic of Tanzania | ... | None | None | None | None | None | None | None | None | None | POLYGON ((33.90371 -0.95, 34.07262 -1.05982, 3... |
2 | Admin-0 country | 1 | 7 | Western Sahara | SAH | 0 | 2 | Indeterminate | 1 | Western Sahara | ... | Unrecognized | Unrecognized | Unrecognized | None | None | Unrecognized | None | None | None | POLYGON ((-8.66559 27.65643, -8.66512 27.58948... |
3 | Admin-0 country | 1 | 2 | Canada | CAN | 0 | 2 | Sovereign country | 1 | Canada | ... | None | None | None | None | None | None | None | None | None | MULTIPOLYGON (((-122.84 49, -122.97421 49.0025... |
4 | Admin-0 country | 1 | 2 | United States of America | US1 | 1 | 2 | Country | 1 | United States of America | ... | None | None | None | None | None | None | None | None | None | MULTIPOLYGON (((-122.84 49, -120 49, -117.0312... |
... | ... | ... | ... | ... | ... | ... | ... | ... | ... | ... | ... | ... | ... | ... | ... | ... | ... | ... | ... | ... | ... |
172 | Admin-0 country | 1 | 5 | Republic of Serbia | SRB | 0 | 2 | Sovereign country | 1 | Republic of Serbia | ... | None | None | None | None | None | None | None | None | None | POLYGON ((18.82982 45.90887, 18.82984 45.90888... |
173 | Admin-0 country | 1 | 6 | Montenegro | MNE | 0 | 2 | Sovereign country | 1 | Montenegro | ... | None | None | None | None | None | None | None | None | None | POLYGON ((20.0707 42.58863, 19.80161 42.50009,... |
174 | Admin-0 country | 1 | 6 | Kosovo | KOS | 0 | 2 | Disputed | 1 | Kosovo | ... | Admin-0 country | Unrecognized | Admin-0 country | Unrecognized | Admin-0 country | Admin-0 country | Admin-0 country | Admin-0 country | Unrecognized | POLYGON ((20.59025 41.85541, 20.52295 42.21787... |
175 | Admin-0 country | 1 | 5 | Trinidad and Tobago | TTO | 0 | 2 | Sovereign country | 1 | Trinidad and Tobago | ... | None | None | None | None | None | None | None | None | None | POLYGON ((-61.68 10.76, -61.105 10.89, -60.895... |
176 | Admin-0 country | 1 | 3 | South Sudan | SDS | 0 | 2 | Sovereign country | 1 | South Sudan | ... | None | None | None | None | None | None | None | None | None | POLYGON ((30.83385 3.50917, 29.9535 4.1737, 29... |
177 rows × 169 columns
continents = world.dissolve(by="CONTINENT").geometry
continents.plot();
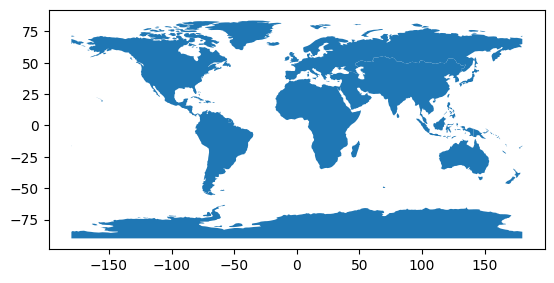
If we are interested in the population per continent, we can pass different aggregation strategies to the dissolve()
functionusing the aggfunc
argument:
https://geopandas.org/en/stable/docs/user_guide/aggregation_with_dissolve.html#dissolve-arguments
continents = world.dissolve(by="CONTINENT", aggfunc=dict(POP_EST="sum"))
continents.plot(column="POP_EST");
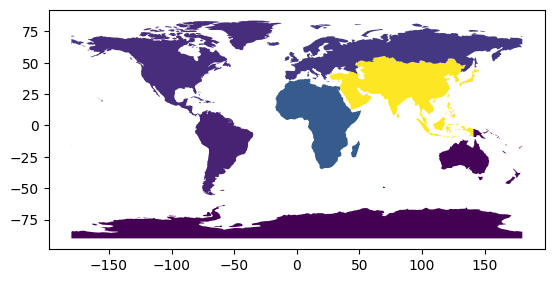
You can also pass a pandas.Series
to the dissolve()
function that describes your mapping for more exotic aggregation strategies (e.g. by first letter of the country name):
world.dissolve(by=world.NAME.str[0], aggfunc=dict(POP_EST="sum")).plot(column="POP_EST")
<Axes: >
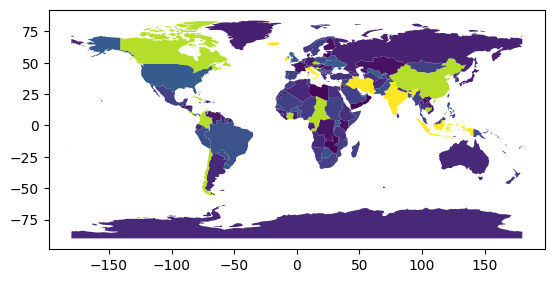
Representative Points and Crow-Fly Distances#
The following example includes code to retrieve representative points from polygons and to calculate the distance on a sphere between two points.
Note
See also https://en.wikipedia.org/wiki/Haversine_formula
See also https://geopandas.org/en/stable/docs/reference/api/geopandas.GeoSeries.distance.html
world = gpd.read_file("https://naciscdn.org/naturalearth/110m/cultural/ne_110m_admin_0_countries.zip")
points = world.representative_point()
fig, ax = plt.subplots()
world.plot(ax=ax)
points.plot(ax=ax, color="red", markersize=3);
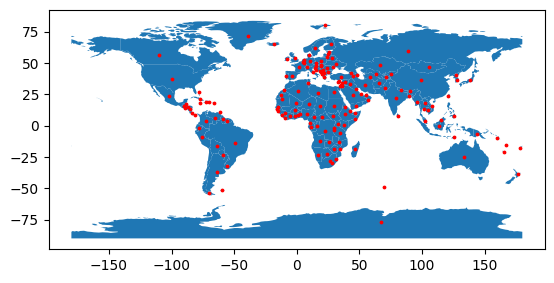
points = points.to_crs(4087)
points.index = world.ISO_A3
distances = pd.concat({k: points.distance(p) for k, p in points.items()}, axis=1).div(
1e3
) # km
distances.loc["DEU", "NLD"]
564.4945392494385
Global Equal-Area and Equal-Distance CRS#
Previously, we used EPSG:3035 as projection to calculate the area of regions in km². However, this projection is not correct for regions outside of Europe, so that we need to pick different, more suitable projections for calculating areas and distances between regions.
for calculating distances: WGS 84 / World Equidistant Cylindrical (EPSG:4087)
for calculating areas: Mollweide (ESRI:54009)
The unit of measurement for both projections is metres.
AREA_CRS = "ESRI:54009"
DISTANCE_CRS = "EPSG:4087"
world = gpd.read_file("https://naciscdn.org/naturalearth/110m/cultural/ne_110m_admin_0_countries.zip")
world.to_crs(AREA_CRS).plot()
<Axes: >
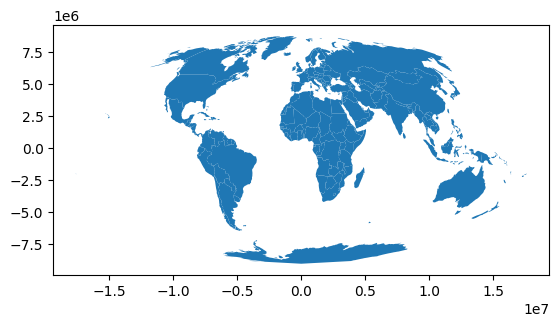
world.to_crs(DISTANCE_CRS).plot()
<Axes: >
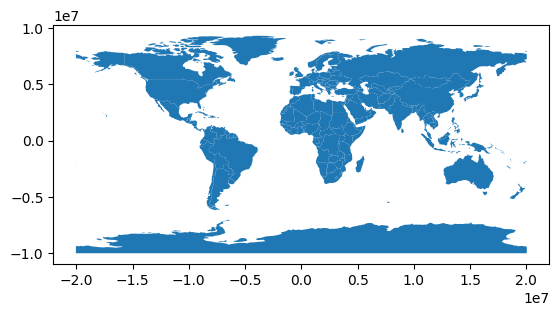
Country Converter#
The country converter (coco) is a Python package to convert and match country names between different classifications and between different naming versions.
Note
See also konstantinstadler/country_converter
import country_converter as coco
Convert various country names to some standard names, specifying source and target classification scheme:
coco.convert(names="NLD", to="name_official")
'Kingdom of the Netherlands'
coco.convert(names="NLD", to="iso2")
'NL'
coco.convert(names="NLD", src="iso3", to="iso2")
'NL'
country_list = ["AE", "AL", "AM", "AO", "AR"]
coco.convert(names=country_list, src="iso2", to="short_name")
['United Arab Emirates', 'Albania', 'Armenia', 'Angola', 'Argentina']
List of included country classification schemes:
cc = coco.CountryConverter()
cc.valid_country_classifications
['DACcode',
'Eora',
'FAOcode',
'GBDcode',
'GEOnumeric',
'GWcode',
'IOC',
'ISO2',
'ISO3',
'ISOnumeric',
'UNcode',
'ccTLD',
'name_official',
'name_short',
'regex']
Gurobi#
Gurobi is one of the most powerful solvers to solve optimisation problems. It is a commercial solver, with free academic licenses.
Note
Using this solver for the group assignment is optional. You can also use other open-source alternatives, but they might just take a little longer to solve.
To set up Gurobi, you need to:
Register at https://pages.gurobi.com/registration/ with your institutional e-mail address (e.g.
@campus.tu-berlin.de
).Follow the getting started guide for your respective operating system at https://www.gurobi.com/documentation/quickstart.html (this includes a guide for retrieving your academic license and installing the software).
In your
conda
environment, installgurobipy
withpip install gurobipy
.